AI-Driven Ad Fraud Detection: How Machine Learning is Safeguarding Digital Advertising
- AI Content Strategist
- Oct 23, 2024
- 4 min read
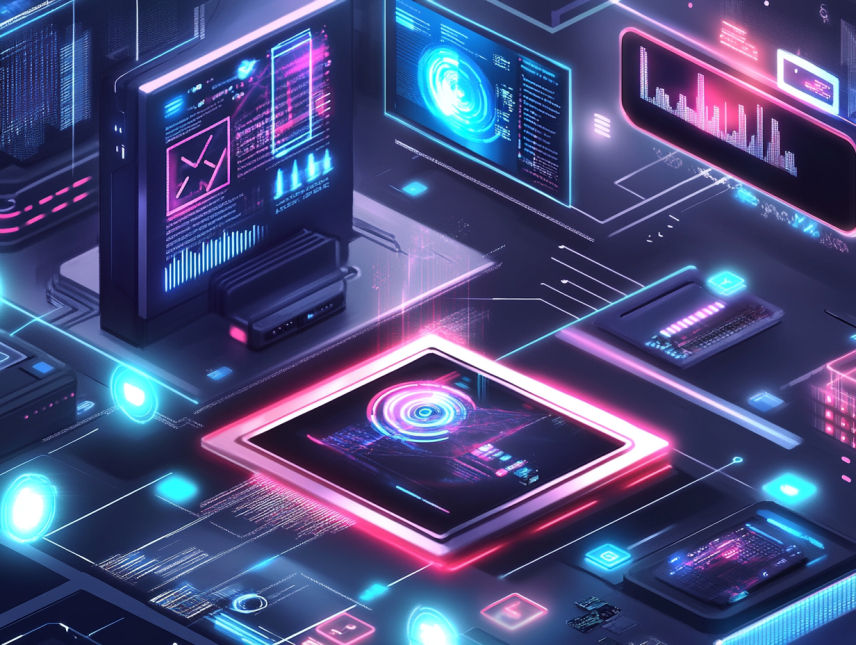
In the fast-paced world of digital advertising, ad fraud remains a persistent threat, costing brands billions of pounds annually. As marketers increasingly turn to data-driven strategies, the need for effective fraud detection has never been more critical. Enter artificial intelligence (AI) and machine learning (ML)—powerful tools that are transforming how brands monitor their advertising campaigns. This article delves into how AI and machine learning algorithms are being harnessed to detect and prevent fraudulent activities, the challenges they face, and the future of digital advertising in this evolving landscape.
The Growing Threat of Ad Fraud
Ad fraud encompasses a variety of deceptive practices that undermine the integrity of digital advertising campaigns. From fake clicks and impressions to bot traffic and ad spoofing, these fraudulent activities not only drain marketing budgets but also distort performance metrics. As the industry shifts towards programmatic advertising—where automated systems buy and sell ad space in real-time—the potential for fraud has grown exponentially. According to recent estimates, global ad fraud could reach as high as $100 billion in the coming years, making effective detection mechanisms essential.
How AI and Machine Learning Combat Ad Fraud
AI and machine learning algorithms offer sophisticated methods for identifying fraudulent behaviour in real-time. Here’s how they work:
Data Analysis: AI systems can analyse vast amounts of data quickly, identifying patterns that might indicate fraudulent activities. By examining user behaviours, IP addresses, and interaction metrics, machine learning models can distinguish between genuine and suspicious traffic.
Anomaly Detection: Machine learning algorithms use historical data to establish a baseline of normal behaviour for ad campaigns. When deviations from this baseline occur—such as an unusual spike in clicks or impressions—AI can flag these anomalies for further investigation.
Predictive Modelling: By leveraging predictive analytics, AI can forecast potential fraud risks before they escalate. This proactive approach allows brands to adjust their strategies and mitigate risks effectively.
Real-World Applications: Brands Leading the Charge
Several brands have successfully implemented AI-driven ad fraud detection systems to protect their digital advertising efforts:
Unilever: This global consumer goods giant has employed AI to enhance its advertising accuracy and efficiency. By utilising machine learning algorithms to monitor ad placements and detect suspicious activities, Unilever has significantly reduced ad fraud risks, leading to a more effective allocation of marketing budgets.
Procter & Gamble (P&G): P&G has harnessed AI and machine learning to refine its advertising strategies. By analysing user data and engagement metrics, the brand can identify fraudulent impressions and clicks, ensuring that its ads reach real consumers.
Facebook: As a leading player in digital advertising, Facebook has invested heavily in AI-driven fraud detection. The platform employs machine learning algorithms to monitor ad interactions and detect fraudulent patterns, helping to safeguard the integrity of its advertising ecosystem.
Challenges in Implementing AI-Driven Fraud Detection
While AI and machine learning offer significant advantages in combating ad fraud, brands face several challenges when implementing these systems:
Complexity of Algorithms: Developing and maintaining effective machine learning models requires technical expertise and ongoing adjustments to keep pace with evolving fraud tactics. Brands must invest in skilled data scientists and engineers to create robust detection systems.
Data Privacy Concerns: The use of AI in ad fraud detection raises potential privacy issues, as brands must navigate the delicate balance between data utilisation and consumer privacy rights. Compliance with regulations such as GDPR can complicate data collection and processing efforts.
False Positives: One of the risks of automated fraud detection systems is the potential for false positives—legitimate clicks or impressions flagged as fraudulent. This can lead to wasted advertising spend and missed opportunities. Continuous refinement of algorithms is crucial to minimise these occurrences.
The Future of Digital Advertising
As AI and machine learning technologies continue to advance, the future of digital advertising is likely to be shaped by enhanced fraud detection capabilities. Brands that invest in AI-driven ad fraud detection systems will not only safeguard their marketing budgets but also improve their overall advertising effectiveness. The increasing sophistication of machine learning models will enable advertisers to better understand their audiences, ensuring that campaigns are more targeted and impactful.
Moreover, as industry standards evolve, collaboration between brands, tech providers, and regulatory bodies will be essential to establish best practices for fraud prevention. This collective effort will help build a more transparent and trustworthy digital advertising ecosystem.
Conclusion
Ad fraud poses a significant challenge to digital marketers, threatening both budgets and brand integrity. However, the integration of AI and machine learning in ad fraud detection offers a promising solution. By harnessing the power of predictive analytics, anomaly detection, and real-time monitoring, brands can effectively combat fraudulent activities and ensure their marketing efforts yield genuine results.
As the digital landscape continues to evolve, embracing AI-driven strategies will be crucial for brands looking to thrive in an increasingly competitive environment. With the right tools and technologies, marketers can safeguard their advertising investments, paving the way for a more effective and transparent future in digital advertising.
Comments